The advent of contemporary AI has opened the door for opportunities to enhance support of healthy aging for older adults through improved health monitoring, efficient assessments, and versatile assistive technologies. With the use of consumer smart devices and wearables expanding, and the cost of these devices decreasing, now is the time for researchers and health systems to leverage these technologies and machine learning approaches to accelerate accessible, personalized care. But challenges still remain that impact implementation and adoption of AI in the healthcare system and the homes of older adults, as well as how and when data collected from various devices can be used. To continue this trend of AI innovation and fully realize potential benefits, it is critical that researchers and investors understand the priorities of older adults and their caregivers, and how these technologies can support healthy aging while minimizing interference with daily living.
On April 3–4, 2025, the a2 Collective, a research program funded by the National Institute on Aging (NIA), part of the National Institutes of Health (NIH), convened its third annual a2 National Symposium to discuss progress, opportunities, and challenges in the design, implementation, evaluation, and commercialization of AI and other emerging technologies for healthy aging and caregiver support. The a2 Collective represents the Artificial Intelligence and Technology Collaboratories (AITC) for Aging Research program, through which NIA plans to award at least $40M over a 5-year period to fund AgeTech pilots with the potential to improve the lives of older adults, including individuals with AD/ADRD, and their caregivers. The symposium was hosted by MassAITC at the Harvard Club of Boston and attended by nearly 200 in-person and more than 50 virtual participants.
AI in cognitive care: enabling early diagnosis and supporting memory
Both home and routine care settings are beginning to use numerous AI-assisted approaches to enable the early diagnosis of cognitive impairment and assist with functions such as memory and word finding. Three speakers and invited panelists discussed the ways in which recent advances in AI and technology can aid the memory of older adults with dementia or cognitive impairment, as well as remaining challenges in implementation, adoption, and ease of use.
Martin Sliwinski, PhD, Penn State University, presented on using mobile technology to monitor cognitive change before noticeable cognitive decline occurs, as primary dementia prevention efforts must begin long before the manifestation of symptoms. By measuring cognitive function much more frequently than typical in-clinic assessments, mobile monitoring platforms can uncover underlying dynamic processes that can help detect subtle changes in cognitive function earlier.
For older adults who already experience memory impairments, Michael Kahana, PhD, University of Pennsylvania, cofounded Nia Therapeutics to develop a biomarker-guided Smart Neurostimulation System (SNS) that electromagnetically stimulates areas of the brain to improve memory. In discussion following these presentations, panelists Carla Bouwmeester, PharmD, RPh, Program of All-Inclusive Care for the Elderly; Chaiwoo Lee, PhD, MIT AgeLab; and Hon Pak, MD, Samsung Electronics, agreed that moving forward, particular focus should be given to (a) ensuring that older adults are not overwhelmed by these technologies, (b) facilitating seamless integration of technologies into the home lifestyle of older adults, and (c) sharing research findings and raw data as broadly as possible to enable further advancements.
Expanding on memory support in the first keynote presentation, Pattie Maes, PhD, Massachusetts Institute of Technology (MIT) Media Lab, shared several projects that aim to support healthy aging with AI systems integrated into smartphones and wearables that help address challenges that come with memory decline. One memory assistant, MemPal, captures pictures of the user’s hands, allowing AI to interpret and document the user’s intended actions. The user can then ask the integrated AI to recall past events, such as where the user left their keys. Memoro, another memory assistant developed in parallel with MemPal, uses speech recognition to document conversations and help the user recall parts of those conversations, and could be used alone or in combination with MemPal to provide augmented support.
Because loneliness among older adults represents a growing concern, MIT Media Lab is also exploring conversational AI applications to support social connectivity. Currently, they are conducting research to inform the design of prosocial AI chatbots that can hold conversations with users about their social relationships and suggest appropriate times to initiate interactions with friends and family. Although interaction with AI can potentially reduce loneliness, several challenges remain, such as individuals who engage in conversations with AI socializing less with humans and some AI models suggesting more antisocial behaviors.
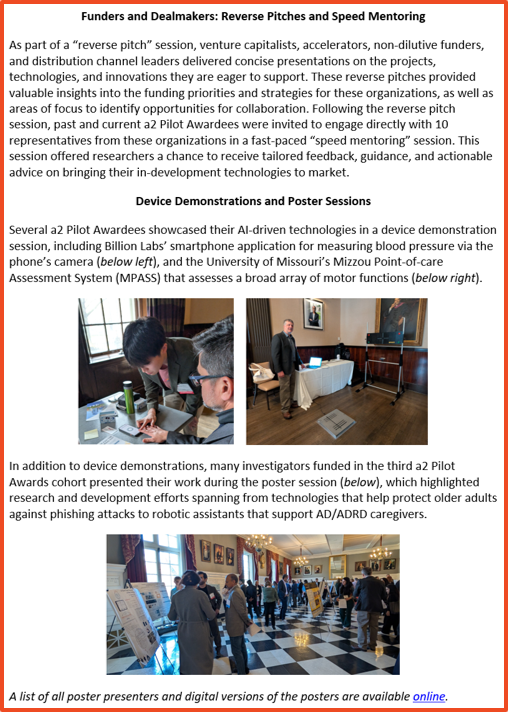
State-of-the-art techniques in AI and machine learning methods for aging and dementia care
As more large, individualized, and complex health datasets become available, researchers must overcome numerous challenges in analyzing these data with AI and machine learning methods, such as developing methods for combining features of multiple datasets and reducing bias in AI outputs. Addressing these challenges can expand the knowledge base of disease progression and guide the design of therapeutics, clinical trials, and ultimately more personalized care plans for older adults.
Conor Walsh, PhD, Harvard University, presented work on machine learning-powered exosuits and rehabilitation gloves that use multimodal sensor data to detect the wearer’s intent and then provide adaptive support for the wearer’s physical actions with greater ease and fluidity. By training individualized models, researchers can provide tailored movement support for individuals who experience movement challenges such as freezing of gait, a common symptom of Parkinson’s disease.
Marzyeh Ghassemi, PhD, MIT, discussed ethical considerations relevant to using visual language models (VLMs), which can follow text prompts to identify subtle but crucial aspects of medical images in order to help clinicians accurately diagnose and treat age-related conditions. VLMs still face several challenges in medical imaging analyses that highlight the need for attending to AI biases, assessing the effectiveness of models in the context of specific settings and population distributions, and determining whether gaps in these AI models’ performance are clinically acceptable.
Following presentations on these cutting-edge methods, invited panelists Alex Zhavoronkov, PhD, Insilico Medicine; Mike Hughes, PhD, Tufts University; and Brian Anderson, MD, the Coalition for Health AI, discussed approaches to improving the accuracy of AI models, including:
• Leveraging personal, individualized data from clinics that generalized AI models can use to learn and train, while also considering the amount of acceptable uncertainty in AI outputs.
• Continuing to provide a “human in the loop” approach, in which experts repeatedly evaluate AI models at various stages of development, implementation, and deployment.
• Ensuring transparency in AI model performance, risk management, and accuracy, which can instill more confidence in clinicians using these models, as well as older adults with safety and privacy concerns.
• Understanding the capabilities and limitations of foundation models, such as OpenAI’s Generative Pre-trained Transformer series, in assisting with specialized tasks to support older adults.
For the second keynote presentation, Jianying Hu, PhD, IBM, outlined how AI can improve the drug discovery process to advance therapeutics for neurodegenerative diseases. Currently, drug discovery is a long and costly process, with only 12% of drug candidates in clinical trials gaining subsequent approval. But recent advances in AI show potential to accelerate the pace of drug discovery across the entire drug development pipeline, from drug target identification to post-approval analysis. For example, researchers can use AI models to generate new small molecules, virtually screen these molecules, and establish initial safety and efficacy profiles before moving toward lab testing. The next challenge in incorporating AI into the drug discovery process is creating models that can represent multiple scales of biology and, importantly, establishing an open research community to drive scalable development, evaluation, and adoption of these models.
Leveraging big health data in the development of AI
AI and machine learning approaches require vast quantities of data for development and training. Fortunately, the increasing capture of digital health data (i.e., “big data”) over the past decades has resulted in large volumes of heterogenous data held by hospital systems, health insurers, wearable device technology companies, and government-funded cohort studies. But the quality of these data, as well as how they are stored and shared among the research community, plays a key role in ensuring the accuracy and readiness of AI systems leveraging them.
In an effort to improve data storage and sharing, NIH’s All of Us Research Program strives to nurture diverse partnerships across the United States to deliver one of the largest and richest biomedical datasets that is broadly available. Jordan Smoller, MD, All of Us, Massachusetts General Hospital, and Harvard University, outlined the extensive data types currently available through All of Us, including survey responses, physical measurements, genotyping, whole genome sequencing, structural gene variants, and electronic health records (EHRs). In addition, the program provides analytic tools for researchers, such as cohort and dataset builders, workflow tools, and various data analysis algorithms.
Although initiatives in large data collection are crucial, connecting large datasets from separate institutions can further expand data sharing efforts. Griffin Weber, MD, PhD, Beth Israel Deaconess Medical Center and Harvard Medical School, has helped develop the Informatics for Integrating Biology and the Bedside (i2b2) platform for integrating EHR, clinical, and trial data into a centralized location. These datasets are then connected through various federated networks that align with specific research objectives. These networks can significantly increase the sample size of studies, allowing researchers to use AI to predict various health conditions and outcomes based on EHR data alone.
Invited panelists Glenn Cohen, JD, Harvard University Law School; Susann Keohane, PhD, IBM; and Sudeshna Das, PhD, Massachusetts General Hospital and Harvard Medical School, continued the discussion on utilizing big health data, revisiting the impact of training AI on biased datasets, as well as regulatory and ethical implications of overlooking AI bias. One way to address AI bias is through data transparency—when data sources are known, then researchers can understand the context from which the data were collected to better mitigate AI bias. Panelists also emphasized the need for maintaining data privacy when collecting and sharing individualized datasets, as well as the complexities of data ownership and how transparent communication about ownership can build trust with study participants and participating communities.
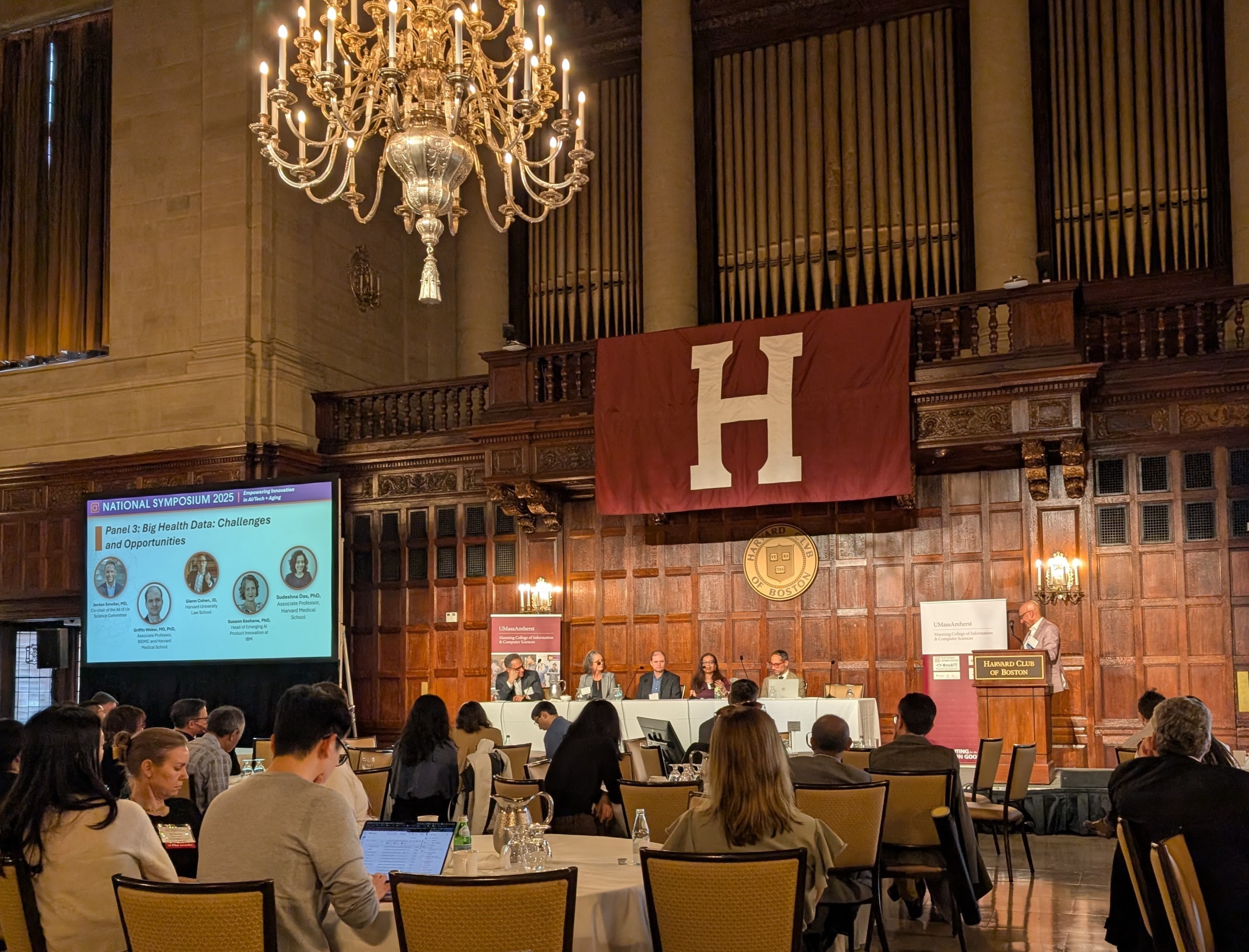
The next a2 National Symposium will take place March 19–20, 2026, in Washington, D.C., and will feature pilot awardees from the fourth and fifth cohorts of funded a2 Pilot Awards projects. Review of applications and selection of projects for the fifth cohort is currently underway. Learn more at a2PilotAwards.ai.
NIA is one of 27 Institutes and Centers of the National Institutes of Health at the U.S. Department of Health and Human Services. The a2 Collective is funded through NIA grants U24AG073094 (the a2 Collective Coordinating Center), P30AG073104 (JH AITC), P30AG073105 (PennAITech), and P30AG073107 (MassAITC).
The full 2025 a2 National Symposium agenda, along with upcoming events, additional resources, and other information, is available online. You can also follow the a2 Collective’s work on LinkedIn and X.